Nvidia, the computer chip designer, has recently experienced a meteoric rise in its share price, propelling the company’s valuation above the coveted trillion-dollar mark. This achievement places Nvidia in an elite club of US companies that includes tech giants Apple, Amazon, Alphabet, and Microsoft.
The surge in Nvidia’s stock was triggered by its latest quarterly results, which were unveiled to the public late on Wednesday. The company announced that it would be ramping up chip production to meet the surging demand in the market.
Nvidia has emerged as a dominant force in the field of chips used in artificial intelligence (AI) systems. The interest in AI reached unprecedented levels following the public release of ChatGPT in November of the previous year, which sent shockwaves well beyond the technology industry.
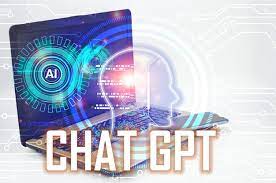
ChatGPT, an AI application that has gained immense popularity, ranging from speech assistance to computer coding and cooking, owes its success to powerful computer hardware, particularly the computer chips manufactured by Nvidia, headquartered in California.
Initially renowned for its graphics processing units (GPUs) designed for enhancing graphics in computer games, Nvidia’s hardware now serves as the foundation for most AI applications. “It is the leading technology player enabling this new thing called artificial intelligence,” says Alan Priestley, a semiconductor industry analyst at Gartner.
Comparing Nvidia’s role in AI to what Intel was to PCs, Dan Hutcheson, an analyst at TechInsights, emphasizes the company’s pivotal position in the AI landscape.
To train ChatGPT, 10,000 of Nvidia’s GPUs were clustered together in a supercomputer owned by Microsoft. Ian Buck, the general manager, and vice president of accelerated computing at Nvidia, highlights that this supercomputer is just one example among many others, both public and undisclosed, that employ Nvidia GPUs for various scientific and AI purposes.
According to a recent report by CB Insights, Nvidia holds around 95% of the GPU market for machine learning.
Nvidia’s AI chips, which are also sold in systems specifically designed for data centers, carry a price tag of approximately $10,000 (£8,000) each, with the latest and most powerful version commanding an even higher price.
So, how did Nvidia establish itself as a central player in the AI revolution? The answer lies in a combination of a bold bet on its own technology and impeccable timing. Nvidia’s CEO, Jensen Huang, one of the company’s founders since 1993, initially focused on enhancing graphics for gaming and other applications.
In 1999, Nvidia developed GPUs to optimize image display on computers. GPUs excel at parallel processing, allowing them to handle numerous small tasks simultaneously. Researchers at Stanford University made a groundbreaking discovery in 2006 when they found that GPUs could accelerate mathematical operations in a way that regular processing chips couldn’t.
This pivotal moment prompted Mr. Huang to invest Nvidia’s resources in creating a tool that would render GPUs programmable, unlocking their parallel processing capabilities for uses beyond graphics.
Nvidia incorporated this tool into its computer chips. While computer game players may not have required this capability, researchers could now perform high-performance computing on consumer hardware, opening up new possibilities for AI development.
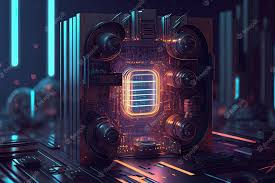
The breakthrough came in 2012 with the unveiling of Alexnet, an AI system capable of classifying images. Surprisingly, Alexnet was trained using just two of Nvidia’s programmable GPUs, significantly reducing the training time from months to a matter of days.
The revelation that GPUs could dramatically accelerate neural network processing spread among computer scientists, who began to purchase GPUs for running this new type of workload. “AI found us,” says Ian Buck.
Nvidia capitalized on this advantage by investing in the development of GPUs better suited to AI applications, as well as software solutions that simplify the utilization of this technology. Over the course of a decade, and with billions of dollars invested, Nvidia’s efforts culminated in the emergence of ChatGPT—a powerful AI capable of delivering eerily human-like responses to questions.
To train and operate its AI models, ChatGPT relies on hundreds of Nvidia GPUs, some purchased directly from Nvidia, while others are accessed through cloud computing services.
Tom Graham, the co-founder and CEO of AI start-up Metaphysic, which creates photorealistic videos using AI techniques, attests that there are no viable alternatives to Nvidia for their work. Graham believes Nvidia is significantly ahead of the competition.
Despite Nvidia’s current dominance, predicting its long-term position in the market is challenging. “Nvidia is the one with the target on its back that everybody is trying to take down,” notes Kevin Krewell, an industry analyst at TIRIAS Research.
Notable semiconductor companies like AMD and Intel provide some competition as they have ventured into dedicated GPUs for AI applications (with Intel joining the fray more recently). Google has its tensor processing units (TPUs) used for search results and specific machine learning tasks, while Amazon has developed a custom chip for training AI models.
Microsoft is reportedly working on an AI chip, and Meta has its own AI chip project. Additionally, emerging computer chip start-ups like Cerebras, SambaNova Systems, and Habana (acquired by Intel) aim to create superior alternatives to GPUs for AI applications by starting from scratch.
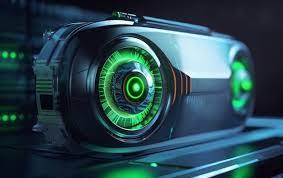
Graphcore, a UK-based company, produces general-purpose AI chips known as intelligence processing units (IPUs), which claim to offer more computational power at a lower cost compared to GPUs. Since its establishment in 2016, Graphcore has received nearly $700 million (£560 million) in funding.
The company’s customers include four US Department of Energy national labs, and it has been lobbying the UK government to incorporate its chips in a new supercomputer project. Nigel Toon, co-founder and CEO of Graphcore, acknowledges the challenge of competing against Nvidia’s dominance.
However, he believes that as AI transitions from cutting-edge experimentation to commercial deployment, cost-efficient computation will become increasingly important.
While competition looms on the horizon, Ian Buck from Nvidia remains undeterred. He emphasizes that the demand for AI is universal, and it is up to other companies to determine how they can contribute to the field.
Nvidia’s formidable position as a driving force in the AI revolution stands unchallenged, but the future holds exciting possibilities and potential disruptions in the rapidly evolving landscape of AI and computer chip technology.